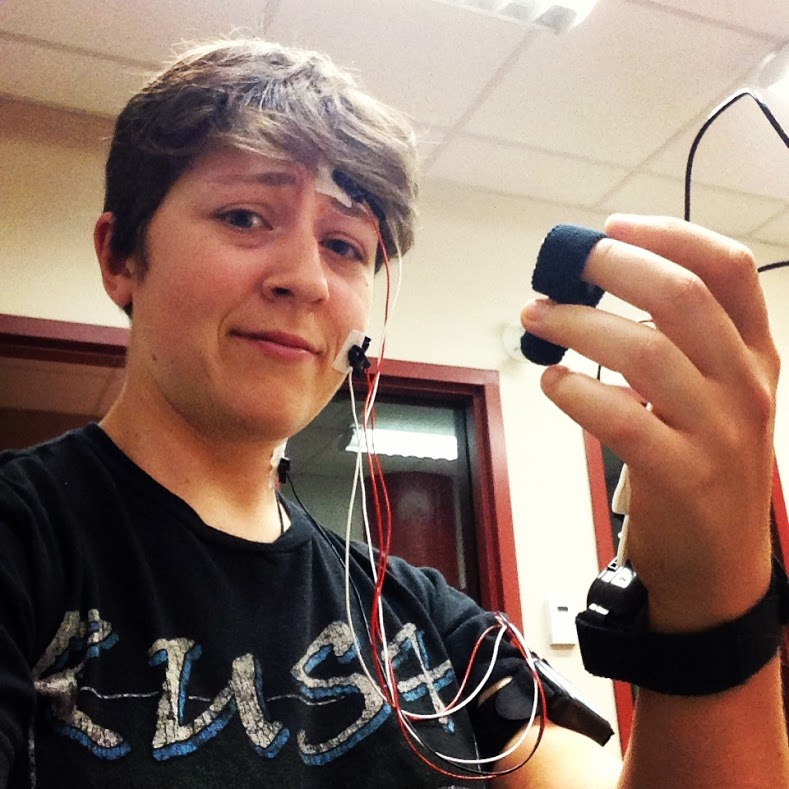
Predicting musically induced emotions from physiological response
Predicting musically induced emotions: linear and neural network models
This is a short blurb about one of the collaborative research projects associated with WaveDNA and the academic papers generated from them. Our research collaborators at Ryerson University’s SMART Lab, Dr. Naresh Vempala and professor Frank Russo, have published a paper recently detailing findings that we will be expanding on and adapting to fit with future WaveDNA products. We describe the study briefly below and provide a link to the paper.
Studies have shown that listeners experience physiological changes during music listening. This study pursues the question of the relationship between the types of physiological responses experienced by a listener and the reported emotion induced by the music they are listening to in real time. Specifically, it addresses the question of whether a listener’s felt emotion can be reliably mapped onto his or her physiological responses during music listening. In a recently published paper in Frontiers in Psychology, the SMART lab and WaveDNA explored this question regarding musical emotion. Five types of physiological features were collected from listeners during music listening, including heart rate, respiration, skin conductance, and facial muscle activity. Listeners heard 12 classical music excerpts from different composers. Neural networks were used to predict the emotional response of listeners from these five physiological features in terms of valence (does it evoke a positive/pleasing emotion, or a negative/grating emotion?) and arousal (does it get your heart racing, or does it help you chill?).
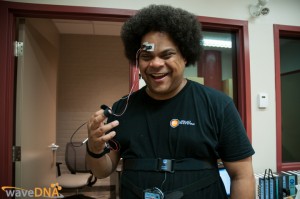
Dave, Co-Founder of WaveDNA obviously having too much fun! Back to designing the future products with you!
Results from this research suggest two things. (1) Physiological responses are powerful indicators of the emotional state music produces in listeners, and (2) a non-linear relationship may be used to map musical emotion onto physiological responses in a reliable way. These findings open up many possibilities not only for further research, but also potentially for powerful new music-making tools or virtual instruments that may guide construction of music production or provide feedback on how a listener might respond to the music you have created. [Imagine, for instance,…]
The research related in the published paper applies to audio-level data, but is being adapted to apply to the finer levels of more detailed features that are available at the MIDI level. The advantage of associating the emotional data with MIDI-level features is that these features may be controllable and could be directly edited and manipulated by software devices not unlike our Liquid Rhythm drum and percussion synthesizer, only including tonal instrument abilities.
http://www.wavedna.com/listening-to-music/
Currently, the SMART Lab/WaveDNA team is processing the results of such a related study that uses MIDI versions of music from popular genres in place of the classical audio pieces employed in the original study. Physiological as well as real-time valance/arousal data was collected from participants, and the source MIDI files have been shredded and processed into tonal Music Molecule features and statistics. The results have been fascinating so far, and promise more insights. Expect to see some intriguing and otherwise unprecedented products and features inspired by this stream of research in the (hopefully) not-too-distant future…
Author: Glen Kappel, Lead Researcher, WaveDNA
Leave a Reply